讲座题目 | AI-Based Air Quality Forecasting Models |
主办单位 | 数理与统计学院 | 协办单位 | 统计与数据计算系 |
讲座时间 | 11月8日 15:30-16:30 | 主讲人 | 金建炳教授 |
讲座地点 | 行政楼1308室 |
主讲人简介 | 金建炳博士,南京信息工程大学环境科学与工程学院校聘教授。2009年考入哈尔滨工业大学,分别获得学士、硕士学位。2015年赴荷兰代尔夫特理工大学留学,2019获得博士学位。同年加入荷兰应用科学院,任助理科学家。2020年入职南信大。金博士主要研究兴趣包括数据同化理论/应用、大气数值模式开发及深度学习在数值模式领域的应用。前期在观测资料偏差校正、观测算子质量控制等数据同化应用领域积累了较多经验,以第一/通信作者发表论文10余篇。 |
讲座内容简介 | The time consumption for air quality predictions is severely constrained by the high computational demands of chemical transport models (CTM) for simulating complex atmospheric reactions. With the rise of AI-based weather prediction models, it has been demonstrated that AI models derived solely from reanalysis data can be highly accurate and efficient. However, the realm of atmospheric chemistry presents greater complexity in terms of dimensions and interactions compared to weather models. To address these challenges, we have developed an AI-based air quality model that incorporates concentrations, emissions, and meteorological factors. This model delivers accurate predictions within a trivial fraction of the time required by traditional CTM models. It then enables rapid large-scale ensemble predictions and emissions inversions, enhancing the feasibility of comprehensive and timely air quality assessments. |
南京信息工程大学金建炳教授为研究生数学建模社师生作学术报告
11月15日下午,南京信息工程大学金建炳教授为研究生数学建模社和学校师生作了题为“AI大模型下空气质量预测模型”的学术报告。数理与统计学院院长王国强主持报告会。
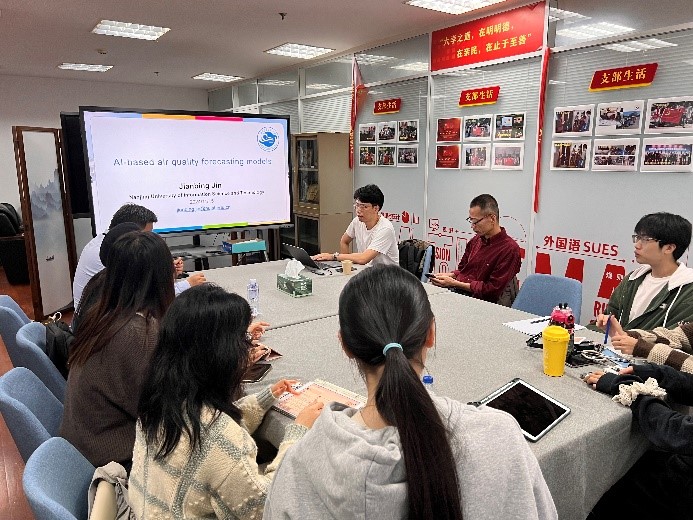
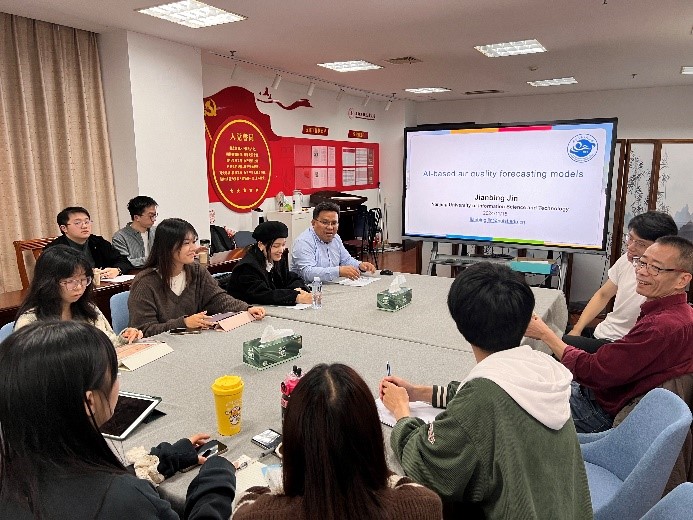
金建炳教授围绕“基于AI大模型的空气质量预测模型”展开介绍了模型的起源、关键指标的筛选及结合数据同化思想的预测过程,并分享了在计算方面的实际应用案例;讲述了关于预测模型的产品迭代过程和各版本的优势;深入解析了数据同化的理论基础,探讨了机器学习与数据同化方法的共性与差异,着重讲解了如何通过大模型的训练来提升预测的准确性与效率,缩短计算时间。
在报告中,金教授结合多个实例,生动展示了大模型在空气质量预测中的具体应用,具有很强的实践指导意义。
此次报告内容丰富、视角广阔,既拓宽了广大师生的思维深度,也深化了大家对数据同化方法在科研中的实际应用理解,提升研究生建模社团建设的专业性和应用性。